Proposing a cnn method for primary and permanent tooth detection and enumeration on pediatric dental radiographs
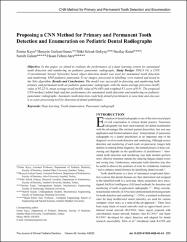
Göster/ Aç
Erişim
info:eu-repo/semantics/openAccessTarih
2022Yazar
Kaya, EmineGüneç, Hüseyin Gürkan
Gökyay, Sıtkı Selçuk
Kutal, Seçilay
Gülüm, Semih
Ateş, Hasan Fehmi
Üst veri
Tüm öğe kaydını gösterKünye
Proposing a CNN Method for Primary and Permanent Tooth Detection and Enumeration on Pediatric Dental Radiographs. (2022). Journal of Clinical Pediatric Dentistry, 46(4), pp. 293-298 https://doi.org/10.22514/1053-4625-46.4.6 Özet
Objective:In this paper, we aimed to evaluate the performance of a deep learning system for automated tooth detection and numbering on pediatric panoramic radiographs. Study Design: YOLO V4, a CNN (Convolutional Neural Networks) based object detection model was used for automated tooth detection and numbering. 4545 pediatric panoramic X-ray images, processed in labelImg, were trained and tested in the Yolo algorithm. Results and Conclusions: The model was successful in detecting and numbering both primary and permanent teeth on pediatric panoramic radiographs with the mean average precision (mAP) value of 92.22 %, mean average recall (mAR) value of 94.44% and weighted-F1 score of 0.91. The proposed CNN method yielded high and fast performance for automated tooth detection and numbering on pediatric panoramic radiographs. Automatic tooth detection could help dental practitioners to save time and also use it as a pre-processing tool for detection of dental pathologies.