A deep learning approach to permanent tooth germ detection on pediatric panoramic radiographs
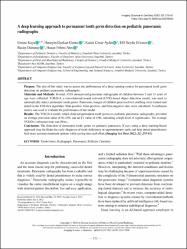
Göster/ Aç
Erişim
info:eu-repo/semantics/openAccessTarih
2022Yazar
Kaya, EmineGüneç, Hüseyin Gürkan
Aydın, Kader Cesur
Ürkmez, Elif Seyda
Duranay, Recep
Ateş, Hasan Fehmi
Üst veri
Tüm öğe kaydını gösterKünye
Kaya, E., Gunec, H. G., Aydin, K. C., Urkmez, E. S., Duranay, R., & Ates, H. F. (2022). A deep learning approach to permanent tooth germ detection on pediatric panoramic radiographs. Imaging Science in Dentistry, 52(3), pp. 275-281 https://doi.org/10.5624/isd.20220050 Özet
Purpose: The aim of this study was to assess the performance of a deep learning system for permanent tooth germ
detection on pediatric panoramic radiographs.
Materials and Methods: In total, 4518 anonymized panoramic radiographs of children between 5 and 13 years of
age were collected. YOLOv4, a convolutional neural network (CNN)-based object detection model, was used to
automatically detect permanent tooth germs. Panoramic images of children processed in LabelImg were trained and
tested in the YOLOv4 algorithm. True-positive, false-positive, and false-negative rates were calculated. A confusion
matrix was used to evaluate the performance of the model.
Results: The YOLOv4 model, which detected permanent tooth germs on pediatric panoramic radiographs, provided
an average precision value of 94.16% and an F1 value of 0.90, indicating a high level of significance. The average
YOLOv4 inference time was 90 ms.
Conclusion: The detection of permanent tooth germs on pediatric panoramic X-rays using a deep learning-based
approach may facilitate the early diagnosis of tooth deficiency or supernumerary teeth and help dental practitioners
find more accurate treatment options while saving time and effort.(Imaging Sci Dent 2022; 52: 275-81)